The AI Winter Phenomenon
Unravel the history of AI Winters, dissect their triggers and consequences, and relate this knowledge to the current AI landscape.
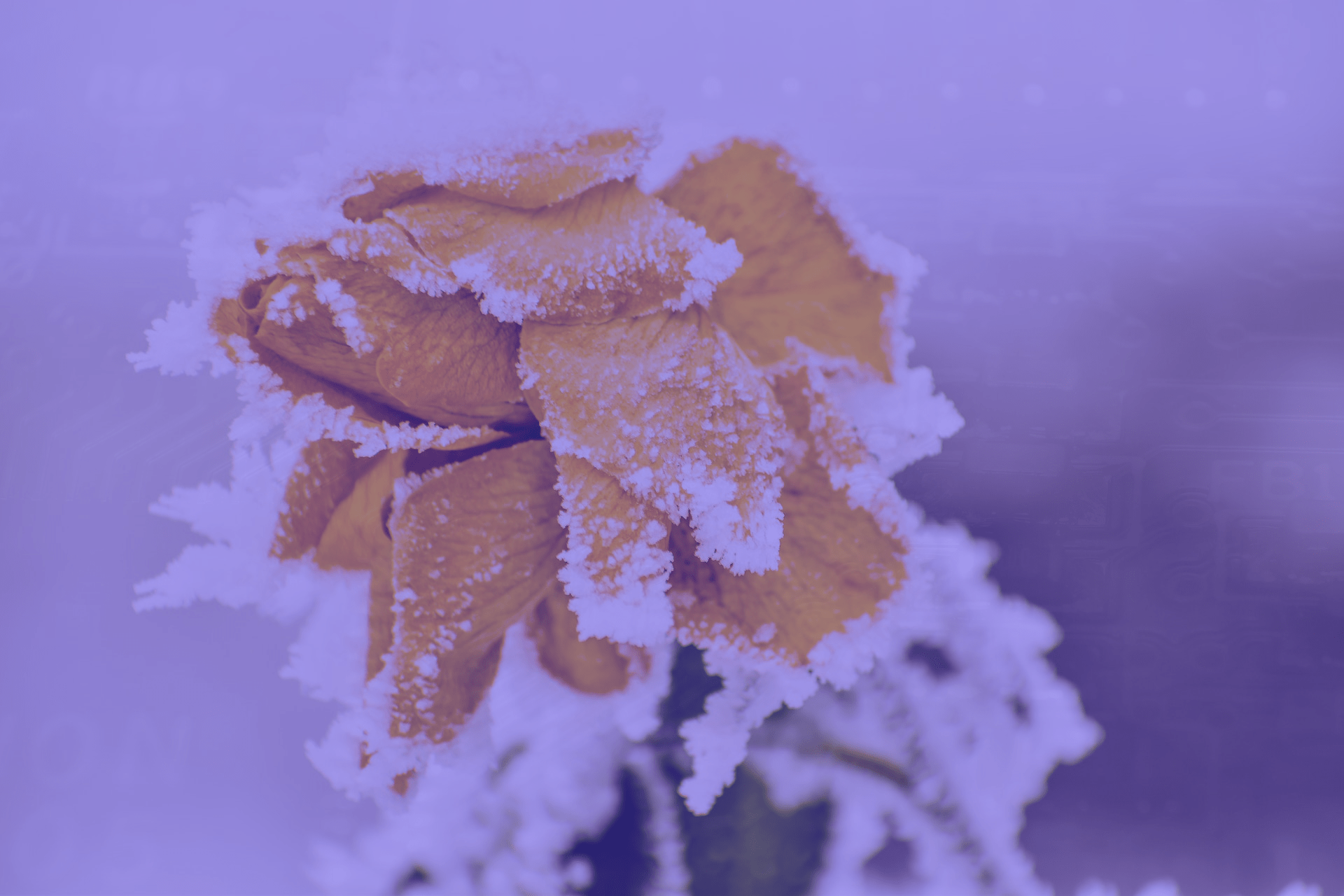
Lessons for Today's AI Landscape
In our January 2005 issue, we published the AI Alphabet. "W" was for "Winter," and we wrote:
W is for Winter. Not the one currently reducing my neighbourhood to near-Siberian cold, but the AI Winter of the late 80s. The phrase was coined by analogy with "nuclear winter" - the theory that mass use of nuclear weapons would blot out the sun with smoke and dust, causing plunging global temperatures, a frozen Earth, and the extinction of humanity. The AI Winter merely caused the extinction of AI companies, partly because of the hype over expert systems and the disillusionment caused when business discovered their limitations. These included brittleness, and the inability to explain their advice at a level of abstraction naïve users could understand.
The term itself, coined in the late '80s, served as a cautionary tale about the cyclical nature of technological advancement and disappointment in the realm of artificial intelligence. The 'Winter' metaphorically froze the ambitions and progress of AI, leading to the extinction of AI companies and a considerable downturn in research and investment.
Fast forward to today, and we're in an era of AI Spring. Innovations in machine learning, natural language processing, and robotics are revolutionizing industries and daily life. Technologies like ChatGPT are breaking barriers in human-machine interaction. Despite this, the specter of AI Winter looms in the background.
Why should we care about a term that originated decades ago, especially when AI seems to be thriving? The answer is simple: understanding the causes and impacts of past AI Winters can serve as a guide for managing current and future developments in AI. It's a lens through which we can evaluate not just the technological advances but also the ethical, societal, and economic implications of AI.
This article aims to unravel the history of AI Winters, dissect their triggers and consequences, and relate this knowledge to the current AI landscape. By doing so, we hope to offer a balanced view that celebrates AI's potential while cautioning against the pitfalls that could lead us into another winter.
AI Winter: A Phenomenon That's More Than Just a Season
When you hear the term "AI Winter," it's easy to picture a scene of desolation where once-promising technologies lie dormant under a blanket of metaphorical snow. The term is as compelling as it is cautionary, and it carries an intricate blend of history, disappointment, and lessons for the future. It was coined in the late 1980s and intentionally draws a parallel with "nuclear winter," a theoretical concept suggesting that a large-scale nuclear war could lead to severely cold weather and dark skies due to firestorms sending soot into the stratosphere. While a nuclear winter speaks to a global calamity, an AI Winter, although less catastrophic, signifies an equally profound crisis within the sphere of technology and innovation.
But what precipitates such a winter in the world of artificial intelligence? A series of interconnected factors usually sets the stage. One of the most glaring culprits is the issue of overhyped expectations. Imagine a startup that promises to revolutionize healthcare through AI, garnering massive investments. But as time passes, it becomes evident that the technology is still in its infancy, incapable of delivering on its grand promises. This breach between expectations and reality can have a cascading effect, causing investors to pull out, leading to financial instability not just for one company but often for the entire sector. The media, once a cheerleader for AI's unlimited potential, turns skeptical, further dampening public enthusiasm.
Technological limitations are another significant factor leading to an AI Winter. In the early days of AI, for instance, computational power was a significant bottleneck. The algorithms that were envisioned required processing capabilities far beyond what was available, turning potentially groundbreaking ideas into little more than theoretical pipe dreams. Even today, with far superior hardware, we face limitations in data quality, algorithmic efficiency, and scalability. These technological shortcomings can lead to project failures, which in turn exacerbate the already growing disillusionment.
Funding cuts are the final nail in the coffin. Whether from governmental organizations, venture capital, or internal corporate budgets, financial support for AI projects often hinges on short-term successes. When these successes aren't realized, the funding dries up. This is particularly damaging for research institutions that rely on grants to explore new AI frontiers. The reduced financial inflow leads to project cancellations, layoffs, and sometimes even the complete dissolution of AI departments.
The consequences of an AI Winter are both immediate and long-lasting. Research stagnates as scientists and engineers find their work either underfunded or entirely defunded. Companies that had bet big on AI face financial ruin, leading to bankruptcies and mass layoffs. Even those that survive often have to pivot away from AI, setting the field back years, if not decades. But beyond these tangible impacts, there's an intangible yet profound effect on morale. Researchers become more cautious, companies become risk-averse, and a general sense of skepticism pervades the field, taking years, if not decades, to fully dissipate.
While the term "AI Winter" has its roots in history, its implications are ever-relevant. Each wave of technological advancement in artificial intelligence brings with it the looming shadow of another potential winter. As we stand on the cusp of AI becoming an integral part of our lives—from healthcare and transportation to how we communicate—it's more crucial than ever to understand what an AI Winter is, what leads to it, and the ripple effects it can have on progress and innovation. This awareness isn't just academic; it's a roadmap that helps us navigate the fragile ecosystem of expectations, technological capabilities, and financial backing that AI currently resides in.
The First AI Winter of 1974–1980: The Dawn of Disillusionment
Picture the 1970s: a time of disco, bell-bottoms, and, believe it or not, unbridled enthusiasm about artificial intelligence. Researchers in lab coats believed they were on the cusp of something revolutionary. The thought was that AI would soon replicate—and even surpass—human intelligence. There were promises of machines that could understand natural language, solve complex equations, and even exhibit common sense. The optimism was palpable, almost infectious, and it seemed like nothing could go wrong.
But then something did go wrong, very wrong. The first significant incident that triggered the onset of the first AI Winter was the publication of the Lighthill Report in the United Kingdom. Commissioned by the British government to evaluate the state of AI research, the report was nothing short of a cold shower. It concluded that AI had failed to achieve its lofty objectives and was unlikely to do so in the foreseeable future. The impact was immediate: government funding for AI research in the UK was slashed, sending a chilling effect through research labs across the country.
Across the Atlantic, the United States was undergoing a similar reckoning. DARPA, the Defense Advanced Research Projects Agency, had been one of the most significant funders of AI research. But growing skepticism about the practical applications of AI led to significant budget cuts. Research projects that once seemed limitless in their potential were suddenly halted, and researchers found themselves scrambling for alternative funding sources, often in vain.
The consequences of this first AI Winter were severe and far-reaching. The most immediate impact was financial. With funding cuts from both public and private sectors, research came to a grinding halt. The slowdown wasn't just a delay; it was a significant setback that pushed the field of AI years, if not decades, behind its projected goals. Many promising projects were left incomplete, their potential untapped.
Beyond the financial ramifications, there was an intellectual and emotional toll as well. Researchers who had once been hailed as visionaries found themselves defending their life's work. The academic community became increasingly skeptical about the prospects of AI, making it difficult for new ideas to gain traction. This skepticism wasn't merely a phase; it lingered for years, shaping the research agenda and tempering expectations for a generation of scientists.
The first AI Winter didn't just freeze academic research; it also put a chill on the commercial landscape. Companies that had ventured into AI technologies found themselves facing a reality that was harsher than they had expected. Several businesses, particularly those that had positioned themselves as frontrunners in AI, felt the blow most significantly.
One notable example was the company Perceptron Inc., founded by Frank Rosenblatt, the inventor of the perceptron learning algorithm. The company promised to revolutionize the field of machine learning and was initially met with significant enthusiasm. However, Marvin Minsky and Seymour Papert of MIT published a book in 1969 called "Perceptrons," which laid out the limitations of perceptron algorithms, particularly their inability to solve problems that weren't linearly separable. The book had a chilling effect on the perception of neural networks and contributed to the skepticism that led to reduced funding.
Another example was the demise of several early natural language processing (NLP) companies. These firms promised machines that could understand and process human languages, a vision that was far too ambitious given the technological limitations of the time. The high failure rate of these ventures only added fuel to the fire of skepticism.
It wasn't just small startups that felt the freeze; larger tech companies with AI divisions also felt the impact. Xerox's Palo Alto Research Center (PARC), famous for its contributions to computer science, saw a significant reduction in its AI research during this period. Projects that once held promise were either scaled down dramatically or scrapped altogether.
AI companies dealing with robotics were another casualty. The expectation had been that we would soon have robots performing a range of tasks from household chores to industrial processes. But the reality was that the technology was nowhere near sophisticated enough to meet these expectations. Companies that had invested heavily in robotics research found themselves facing a dead end.
The narrative of these companies serves as mini-tragedies within the larger drama of the first AI Winter. They are tales of ambition, promise, and ultimately, disappointment. Each company that folded or shifted its focus away from AI represented a loss of potential and a setback for the field. The ripple effects were felt far and wide, affecting investor confidence, job markets, and even educational programs related to AI.
This first AI Winter served as a sobering lesson in humility. It illustrated the chasm that can exist between ambition and reality, between expectation and execution. But more than anything, it instilled a sense of caution that would define the field of artificial intelligence for years to come. As new waves of technology emerged in the years that followed, this caution served as both a safeguard and a reminder of the perils of overreach.
The Second AI Winter (1987–1993): The Chill Returns
The late 1980s seemed like a renaissance period for artificial intelligence. After the disillusionment of the first AI Winter, the focus had shifted toward a highly specialized and seemingly practical application of AI: expert systems. In stark contrast to the broad and often nebulous goals of early AI initiatives, expert systems had a laser focus. They were designed to mimic human expertise in extremely specialized fields, from medical diagnostics to stock trading. Industry giants like IBM, Digital Equipment Corporation (DEC), and a swath of startups like IntelliCorp and Teknowledge were investing heavily in this promising subfield. There was a palpable sense that AI had moved from being a research curiosity to becoming a commercially viable technology.
However, the promise of these early years quickly gave way to another chilling period known as the second AI Winter. This phase was characterized by a sequence of setbacks and disappointments, which led to another significant contraction in both interest and investment in the AI field.
Firstly, the limitations of expert systems became increasingly apparent. These systems were often described as 'brittle' because of their inability to adapt or generalize their understanding to new situations or contexts. For example, a medical diagnostic system could be extremely proficient when dealing with conditions it had been programmed for but would fall short when confronted with an uncommon or complicated case. This brittleness turned into a major point of criticism and led to declining confidence in the technology.
Another issue was the systems' 'opacity' or their inability to explain their decisions and reasoning processes in a comprehensible manner. This was particularly problematic in fields where interpretability was crucial. For instance, in medical or legal settings, professionals are trained to make decisions based on a clear rationale; they could not simply rely on the output of an 'opaque' system. The inability of these expert systems to provide understandable explanations for their decisions further eroded trust in this technology.
But technical limitations were just one part of the story. The timing of the expert system boom coincided with economic challenges, including market crashes and recessions. Black Monday in 1987 was a particularly significant event that led to increased economic conservatism and a subsequent reduction in risky investments, like AI technologies. Companies, now operating in an economically sensitive environment, became more risk-averse, cutting funding to AI projects deemed too experimental or unproven.
As a result, the second AI Winter had devastating consequences for the AI community. High-profile casualties included companies like IntelliCorp and Carnegie Group, which saw drastic reductions in their valuation. IntelliCorp, which had been a major player in the expert systems arena, faced dwindling revenues and market share. Carnegie Group wasn't spared either; the company faced financial difficulties that led to its acquisition at a bargain price, a sad exit for a one-time industry leader.
Large corporations with AI-focused departments or subsidiaries also felt the squeeze. IBM, which had been a significant player in the development of expert systems, was forced to scale down its AI ambitions dramatically. Projects were either canceled, or budgets were slashed, leading to layoffs and a reduced focus on AI research and development.
Investment from venture capital firms and government grants dried up. Research institutions and academic departments faced funding cuts, forcing a pivot or downsizing of AI research programs. The resulting atmosphere was one of caution and skepticism. Researchers, who had once been optimistic about the transformative potential of expert systems and AI, found themselves defending their work and fighting for a shrinking pool of resources.
The second AI Winter was a humbling experience for everyone involved. It served as a stark reminder that technological potential alone was not enough to sustain a field. Real-world applicability, economic viability, and the ability to meet or manage expectations are equally crucial. As the AI community emerged from this challenging period, these hard-learned lessons would go on to shape the strategies, expectations, and caution exercised in subsequent years, preparing the field for the resurgence we see today.
Near-Misses and Mini AI Winters:
Artificial Intelligence has often been viewed through a binary lens: periods of extreme hype followed by so-called AI Winters. However, the landscape is more nuanced, featuring intervals that can be best described as 'near-misses' or 'mini-winters.' These are times when the field experienced slowed growth or reduced enthusiasm but didn't dive into a full-fledged winter. Two significant periods of such near-misses stand out: the aftermath of the Dot-Com bubble in the late 1990s and a quieter period in the mid-2000s.
The Dot-Com bubble burst of the late 1990s affected the entire tech industry, and AI was no exception. Investors who were previously willing to pour money into anything with a '.com' in its name suddenly became far more cautious. Startups like Kozmo.com, which had ventured into automated logistics and delivery—an early application of AI in supply chain management—went bankrupt. Similarly, firms like Boo.com, which aspired to create AI-driven personalized shopping experiences, also failed. Yet, it's crucial to note that these failures were part of a broader tech industry slump, not specific to AI. The general sentiment was one of caution towards all things tech, rather than a loss of faith in AI's potential.
The mid-2000s brought another period of slowed momentum in AI. The initial buzz around the Internet had settled, and the industry was searching for the 'next big thing.' Companies like Cycorp, which had been working on a general AI system known as Cyc since the 1980s, struggled to maintain momentum. Similarly, chatterbot projects like SmarterChild, which aimed to provide a conversational interface for various online services, saw limited commercial success. However, the industry's focus was shifting towards what AI could practically offer. Instead of sweeping projects aiming to replicate human intelligence, efforts were more targeted, focusing on natural language processing, recommendation systems, and data analytics.
Several factors helped the AI field avoid a full-blown winter during these periods. One of the key reasons was the steady, albeit slower, pace of technological advancements. During the late 1990s, Support Vector Machines (SVM) and other machine learning algorithms were developed, laying the groundwork for future AI applications. In the mid-2000s, we saw incremental but meaningful advancements in natural language processing and computer vision, partly fueled by increased computing power and the availability of large datasets. These weren't groundbreaking advancements, but they kept the wheels of progress turning.
Another protective factor was the diversification of AI into various sectors. In the mid-2000s, AI technologies began to find applications in areas as diverse as healthcare diagnostics, automated trading systems in finance, and even in video games for creating more intelligent and challenging opponents. Companies like Nuance Communications made strides in healthcare-focused speech recognition, while firms like Blue River Technology started using computer vision in agriculture. This diversification meant that even if one area faced setbacks, the entire field wouldn't come to a standstill. The potential for AI was broad enough to absorb these shocks, and this distributed risk helped maintain a baseline level of activity and interest in AI.
These near-misses serve as both cautionary tales and testimonies to the resilience of the AI field. They remind us that the road to AI's future is not a straight, upward trajectory but a path filled with ups and downs. While these periods didn't trigger full-scale winters, they did induce a level of introspection and caution that helped steer the field. They forced AI practitioners, investors, and policymakers to temper expectations, refine focus, and develop more realistic, achievable goals, making the field more robust and better prepared for the challenges of the future.
The Current State of AI:
The resurgence of interest and investment in artificial intelligence (AI) is nothing short of phenomenal, and at the core of this renaissance lies machine learning, a subset of AI that provides systems the ability to automatically learn and improve from experience. Companies big and small are delving deep into machine learning to solve complex problems, automate tedious tasks, and create innovative products and services. This is not just a trendy focus; it's a fundamental shift in how we approach computation and data analysis.
Within machine learning, deep learning techniques have been particularly transformative. Utilizing neural networks with multiple layers, deep learning models analyze different forms of data to recognize patterns and make decisions. Google's DeepMind has made remarkable strides in healthcare, diagnosing conditions from eye scans with accuracy comparable to human experts. The platform has even ventured into protein folding, a complex biological problem, providing solutions that could revolutionize drug discovery.
But Google is not alone in the race. Microsoft's Azure Machine Learning provides enterprises with scalable solutions, enabling them to build, train, and deploy machine learning models efficiently. Its capabilities extend from text analytics and recommendation services to more advanced applications like anomaly detection in IoT devices. These machine learning solutions are not confined to tech giants; they are increasingly accessible to startups, researchers, and even hobbyists, thanks to open-source libraries and community contributions.
Natural Language Processing (NLP) is another subfield of AI that has witnessed significant advancements. This technology focuses on the interaction between computers and human language. Gone are the days when chatbots offered rigid, scripted responses. Modern NLP models can understand context, sentiment, and even nuances like sarcasm. One of the most groundbreaking developments in this area has been OpenAI's GPT-3, which set new standards for machine-generated text. From summarizing legal documents to creating Python code, GPT-3 demonstrated a versatility that was previously unimaginable.
The evolution didn't stop there. ChatGPT-4, the latest iteration, brings a host of improvements, including a built-in Python interpreter. This unique feature enables the chatbot to execute Python commands in real-time, offering a level of interactivity previously unseen. Imagine a customer support chatbot that doesn't just provide troubleshooting steps but actually executes diagnostic tests through Python commands, all within the same conversational window. The possibilities are endless and stretch across domains like education, healthcare, and even entertainment.
Robotics is another sector where AI has been a game-changer. The robots of today are not just mechanical arms confined to factory floors; they are increasingly sophisticated machines capable of complex tasks. Companies like Boston Dynamics are pioneering this space with robots that can navigate rough terrains, climb stairs, and even dance. Their robot, Spot, is already being deployed in industrial settings for inspections, data collection, and security.
On the transportation front, Tesla is making significant strides towards fully autonomous vehicles. The advanced driver-assistance systems in Tesla cars, powered by AI algorithms combined with in-house chips specifically built for AI offer self-driving, adaptive cruise control, and even "Summon," where the car comes to the owner in a parking lot. These are not isolated experiments but features available to consumers today, marking a significant step towards the future of fully autonomous transportation. The dreams of the past are coming true in the present.
AI's current surge is not just technological; it has significant economic implications. The automation of tasks across sectors is creating new job categories. Companies like UiPath are specializing in Robotic Process Automation (RPA), creating roles for RPA developers and consultants. Similarly, the demand for data scientists, machine learning engineers, and AI ethics specialists is on the rise. While job creation is a positive aspect, there is also the undeniable reality of job displacement. Automation is reducing the need for human intervention in repetitive tasks, leading to debates on workforce re-skilling and the future of work.
While AI's capabilities are awe-inspiring, they come with a set of ethical challenges that society is still trying to comprehend. Data privacy is a significant concern, especially with AI's ability to analyze and make decisions based on vast datasets. Algorithmic biases, where AI systems inadvertently adopt the prejudices present in their training data, are leading to unjust and, at times, discriminatory decisions. These issues have given rise to an entirely new field of study—AI ethics. This discipline aims to address the ethical implications of AI, from data handling and job displacement to more dystopian concerns like autonomous weaponry and mass surveillance.
The ethical considerations are so pressing that academic institutions are introducing courses in AI ethics, and companies are hiring AI ethicists. As AI continues to integrate into the fabric of daily life, these ethical considerations are not just philosophical debates but real-world issues that require immediate attention and resolution.
As we revel in this period of AI advancement, it's crucial to maintain a balanced perspective. The lessons from past AI Winters serve as a cautionary tale, reminding us that while technological advancements are exciting, they must be approached responsibly. The current state of AI presents a blend of opportunities and challenges that require a harmonious balance of innovation, ethical consideration, and societal impact.
Could Another AI Winter Happen? Balancing Boom, Bust, and Geopolitics
The history of AI is characterized by cycles of intense optimism followed by periods of disillusionment, known as "AI Winters." As we experience another AI boom, questions about the sustainability of this growth naturally arise. Could another AI Winter be looming? Several factors could either precipitate or prevent another downturn in the AI landscape.
The overvaluation of AI companies is a concern that cannot be ignored. The AI startup ecosystem is bustling with activity, but there's a growing sentiment that the market might be overheated. Companies claiming to use AI for everything from automated customer service to medical diagnostics have received valuations that might not align with their current capabilities. If these companies fail to deliver on their promises, a loss of investor confidence could trigger a new winter.
Geopolitical factors also come into play, especially with the U.S. advanced chip export ban on China. This move restricts China's access to cutting-edge AI chips produced by American companies. Such geopolitical tensions could stifle innovation and cooperation in the AI sector, potentially leading to stagnation or decline. However, China is not sitting idle; the announcement of a $40 billion chip fund to counter the U.S. chip ban indicates a strategic move to become self-reliant in this crucial technology. This kind of focused investment could either insulate them from a potential AI Winter or create a parallel ecosystem entirely. The latter seems more likely with the level of investment being made.
However, we should also mention that the threat of another AI Winter is not just limited to geopolitical factors. The current AI boom is fueled by a combination of factors, including the availability of large datasets, increased computing power, and advancements in machine learning algorithms. If any of these factors were to change, it could have a significant impact on the AI landscape. For example, if the availability of large datasets were to decline, it could lead to a slowdown in AI research and development. Similarly, if the rate of advancement in machine learning algorithms were to slow down, it could lead to a reduction in the number of practical applications of AI. These factors are not mutually exclusive; they are interconnected and could have a cascading effect on the AI ecosystem.
The availability of large datasets is definitely not guaranteed. OpenAI announced a bot crawler similar to Googlebot earlier this year. By modifing the robots.txt of a website, webmasters have the ability to block OpenAI from crawling their sites and from ChatGPT being able to access it to answer questions. This could lead to a reduction in the availability of large datasets, which could have a significant impact on AI research and development. We are already hearing that large corporations are reevaluating the large datasets they have made available to the public. Twitter just announced they were not allowing their site to be crawled. This could be a sign of things to come.
Shifting work dynamics due to widespread work-from-home arrangements, along with rising inflation and fears of another recession, also add uncertainty and increase the likelyhood of another AI Winter. Economic downturns typically result in reduced spending on research and development, which could have a domino effect on AI funding and innovation. These macroeconomic variables could be catalysts for a reduction in AI activities, potentially signaling the onset of another winter.
Furthermore, ethical and societal challenges associated with AI are becoming increasingly prominent. From algorithmic bias and data privacy issues to the broader implications for employment and social equality, these challenges are complex and multi-faceted. Zoom, had controversy earlier this year when they announced they would use customer data to train AI models. By using many AI systems today, you are opting into your data being used to train AI models. This has caused many corporations to ban the use of AI systems outside their control. Failure to address these issues responsibly could lead to public and governmental backlash, reducing funding and interest in AI technologies.
Despite these concerns, several robust counterarguments suggest that another AI Winter is far from guaranteed. The level of investment from major tech companies like Google, Amazon, and Microsoft is unprecedented. These companies are not merely pumping money into the sector but are also spearheading research initiatives to tackle both technical and ethical challenges. This sustained investment could act as a stabilizing force, mitigating the risks of a major downturn.
Moreover, the diversity of AI's practical applications today serves as another buffer against a potential winter. Unlike earlier periods of AI development, which were often confined to academic or specialized industrial applications, AI today has permeated almost every sector. From automating supply chain logistics to assisting in medical diagnoses and driving advancements in energy sustainability, the technology's broad utility makes it less susceptible to a complete collapse in interest or funding.
While the risk of another AI Winter is not zero, the landscape is far more nuanced than before, with complex geopolitical and economic factors at play. A balance of caution and optimism is essential as the field continues to evolve. The current state of AI reflects both its extraordinary promise and the considerable challenges it faces, a duality that will likely define its trajectory for years to come.
Lessons from Past Winters for the Present
The tumultuous history of artificial intelligence, characterized by soaring highs and dismal lows, offers a reservoir of lessons that are critical for the current state and future trajectory of the field. These lessons, gleaned from periods known as AI Winters, serve as both guiding principles and cautionary tales. As we venture further into an era marked by astonishing advancements in AI, it becomes ever more crucial to heed these historical lessons to ensure a balanced, responsible, and sustainable future for AI.
The first lesson that stands out is the crucial importance of managing expectations. As discussed earlier, the First AI Winter from 1974-1980 was significantly triggered by inflated promises. Companies like Perceptron Inc. ventured into complex fields like machine vision without adequate technological backing, leading to disappointment and a subsequent loss of investor confidence. The Second AI Winter (1987-1993) followed a similar trajectory, where expert systems were touted as the next big thing, but their limitations in terms of brittleness and inability to explain their reasoning led to disillusionment. It's imperative to remember these cases when evaluating the claims of modern AI companies. Overhyping the capabilities of AI technologies, such as claiming they can replace all human-driven diagnostic methods in healthcare or fully automate complex creative tasks, can set us up for another period of disappointment and declining investment.
Another lesson that emerges strongly from the past is the need for transparency and ethical considerations in AI. During the Second AI Winter, one of the significant criticisms of expert systems was their "black box" nature, where the process of decision-making was not transparent. This issue is even more relevant today, as more complex and impactful AI systems are deployed in critical areas like healthcare, criminal justice, and finance. As we've seen with modern challenges around the ethics of facial recognition technology and algorithmic bias in loan approvals, a lack of transparency can erode public trust and lead to regulatory backlash. Ethical lapses can not only cause immediate harm but also contribute to a climate of skepticism that could trigger another winter. Companies and researchers must prioritize ethical guidelines and transparency to maintain public trust and ensure the technology’s long-term viability.
Finally, the role of incremental progress should not be underestimated. History shows us that AI doesn't usually advance through sudden leaps but rather through a series of smaller, yet significant, steps. For example, the transition from ChatGPT-3 to ChatGPT-4 was not marked by a revolutionary change but by incremental improvements that significantly enhanced its capabilities, including the addition of a Python interpreter. Similarly, the sustained growth in AI after periods of stagnation, such as the late 1990s and mid-2000s, was often due to incremental advancements in machine learning algorithms, data analytics, and hardware capabilities. These smaller victories cumulatively contribute to the field's resilience, enabling it to weather periods of skepticism or reduced funding.
Today's AI landscape is marked by both unprecedented opportunities and complex challenges, from technological and economic to ethical and societal. As AI technologies become more integrated into the fabric of our lives, the lessons from its past become increasingly relevant. By carefully managing expectations, putting a premium on transparency and ethics, and valuing the role of incremental progress, we can navigate the present complexities. Doing so will not only help prevent another AI Winter but also ensure that AI develops in a manner that is beneficial to society at large.
Navigating the Future: Cautious Optimism Rooted in Historical Lessons
As we stand at the cusp of a new era in artificial intelligence, it's essential to approach the future with both caution and optimism. The technology's potential to revolutionize multiple facets of our lives, from healthcare and education to governance and entertainment, is truly staggering. However, this enthusiasm must be tempered by the lessons learned from past AI Winters, periods of stagnation and reduced funding that followed overblown expectations and technological limitations.
Managing expectations is not about stifling innovation but about creating a sustainable pathway for growth. The past teaches us that overhyping AI's capabilities can lead to widespread disillusionment, impacting the entire ecosystem from researchers to investors. We must strive for a balanced view that aligns public and investor expectations with what the technology can realistically achieve at each stage of its development.
Transparency and ethical considerations are not just buzzwords; they are essential elements in the responsible development and deployment of AI. The complexities and societal impact of AI require a nuanced approach that prioritizes ethical imperatives alongside technological advancements. As we integrate AI further into critical areas of human life, maintaining transparency and ethical standards will be crucial for sustaining public trust and avoiding regulatory setbacks.
Moreover, the value of incremental progress cannot be overstated. While breakthroughs are exciting, they are few and far between. More often, it's the small, incremental steps that drive the field forward, providing the resilience to weather periods of reduced funding or waning interest. The transition from ChatGPT-3 to ChatGPT-4, marked by subtle but impactful enhancements, serves as a recent example of how incremental improvements can have a transformative effect on technology and its applications.
As we navigate this exciting yet challenging landscape, the lessons from past AI Winters offer invaluable guidance. By adopting a cautious yet optimistic approach, we can foster an environment where AI not only reaches its full potential but does so in a manner that is ethically sound and socially beneficial. The future of AI is a tapestry yet to be woven, but with the right measures, it can be one that enriches the fabric of human society.
Books for Further Reading:
H.P. Newquist's book "The Brain Makers" is still just as relevant today as it was when we first wrote about AI Winter. This book offers an look into the business aspects of AI's history, especially focusing on the period known as the AI Winter. Newquist delves into the rise and fall of AI companies, providing anecdotes and analyses that help readers understand the complexities of the AI business landscape. The book remains a compelling read for anyone interested in the commercial challenges and triumphs of AI over the years.
Another highly recommended book is "Artificial Intelligence: A Guide to Intelligent Systems" by Michael Negnevitsky. This book provides a comprehensive overview of AI technologies, their applications, and their historical context. It serves as both an introduction for those new to the field and a detailed guide for more experienced readers.
"Machines of Loving Grace: The Quest for Common Ground Between Humans and Robots" by John Markoff is also worth a read. This book explores the ethical and societal implications of AI and robotics, a topic that has gained increasing importance in recent years. Markoff offers a balanced view, discussing both the transformative potential of AI and the ethical dilemmas it poses.
"The Master Algorithm: How the Quest for the Ultimate Learning Machine Will Remake Our World" by Pedro Domingos offers a more contemporary take. The book explores the ongoing search for a 'master algorithm' that could potentially revolutionize the AI field. While this may sound like a lofty goal, the book is grounded in current research and provides insights into the cutting-edge developments that are shaping the future of AI.
Each of these books offers a unique lens through which to view the field of artificial intelligence. Whether you're interested in the business aspects, the ethical considerations, or the future possibilities, these reads offer something for everyone looking to deepen their understanding of the complex and ever-evolving AI field.